Content analysis is a key component of a successful marketing strategy, but it’s extremely time-consuming and resource-intensive. Using AI for content analysis is a way to uncover hidden patterns and deliver actionable insights, and something every marketer should be doing.
Now, even small- and medium-sized businesses can gain access to knowledge that before might have only been available to large corporations. AI can analyze customer sentiment and campaign engagement, and even predict what type of content you should make next.
AI content analysis can automate processes like A/B testing and SEO optimization, which gives you more time and energy to focus on strategy. Keep reading to learn about how you can implement this tool in your business, and best practices and tools for global brands.
Here’s our recap:
The role of AI in content performance analysis
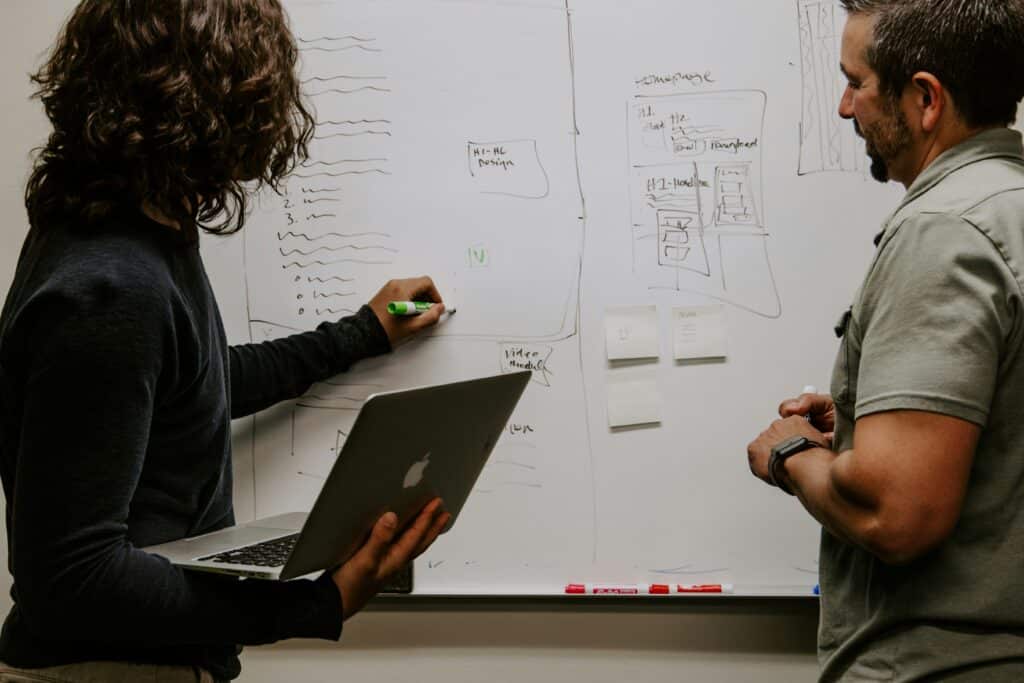
As marketers, we know how critical content performance analysis is. Effectively analyzing your content’s performance helps ensure your content aligns with your marketing objectives and keeps your approach user-centric. It can even help you brainstorm new content for the future based on what’s working or not right now.
However, manual content analysis is extremely time and resource-intensive, as it involves manually reviewing and analyzing vast quantities of data. Not only is this time-consuming, but the sheer amount of data can be overwhelming and even prevent you from gaining meaningful insights, as some patterns in the data may not be obvious to the naked eye.
That’s where AI comes in. Its recent proliferation in a wide variety of marketing tools helps your content marketing be more efficient, accurate and scalable. Especially for small- and medium-sized businesses, analyses that may have once only been available to massive corporations with a ton of resources are more easily accessible than ever.
AI systems can not only quickly process vast amounts of data, but also provide actionable insights directly based on that data. It can even pinpoint customer sentiment and social engagement by combing through posts on different platforms. All of this insight, at a speed significantly faster than any human could do, gives companies the agility to adjust their strategies in real time.
Other concrete ways in which AI content performance analysis can boost your marketing efficacy include:
- Content testing and SEO strategy. With AI, you can run A/B testing and optimize content in real-time based on audience response.
- Analysis of current search trends and keyword performance. AI tools provide suggestions to elevate your content’s visibility.
See also: Generating quality global content at scale using AI tools
Important steps to effectively implement AI content analysis into your marketing strategy:
1. Create a customer persona
As AI operates based on statistical models, not actual “intelligence” like a human, you need to give it specific data and parameters that allow it to most accurately analyze and provide suggestions for your business. One of the most important parameters you can create and give to an AI is your customer persona.
Creating a customer persona specifically for AI content analysis might require you to provide more data and do some more research, but it will greatly enhance your analysis results. Here’s how:
- You can use a generative AI tool like ChatGPT to generate starting ideas for your customer persona.
- Gather customer data from different channels like CRMs, social listening, and surveys.
- Once you’ve assembled your information, provide ChatGPT demographic facts of your customer profile, like age, job title, industry, company size, geography, responsibilities, goals, challenges, content preferences, and their buying journey.
- After generating the initial persona, you can have a “conversation” to better refine the profile. For example, telling it to change a specific concern to something more relevant, or prioritizing a certain channel or funnel over another.
We recommend that if you’re using an open AI model to use a fake brand name in your training, and always have a human look over and refine anything created by a generative AI tool for accuracy.
2. Collect data
AI content analysis is only as good as the data you provide it, so be sure to collect data from as many sources as necessary. AI tools can analyze:
- Text data: articles, social media posts, blog posts
- Visual media: images and videos used in content marketing
- Behavioral data: user behavior on websites, social media platforms, and other channels
- Demographic data: users’ age, gender, location, and other demographic factors
- Performance data: metrics like page views, engagement rates, click-through rates, and conversion rates
Beyond your obvious sources of data like email marketing platforms and CRMs, you can leverage different tools that streamline data collection: platform APIs, web scraping tools, and analytics tools like Sprout Social. Depending on what tool you’re using, some can even be linked to certain datasets and be fed data as it’s being created. Make sure that the metrics you’re collecting align with your specific content goals.
3. Clean and organize
Once you collect the data you need, you have to clean it and prepare it for AI analysis. Remove duplicates, missing values, and any irrelevant data you may have pulled. Standardize all formats, like dates and units of measurement. If you have data from multiple sources that are on the same topic, combine that data into a single dataset. Finally, label and categorize your data (like by campaign name or content type).
You also need to make sure that the data you’re providing is in the optimal format for AI analysis. The optimal format depends on the type of data you’re using:
- Tabular, structured data is best as a CSV, JSON, or Excel file.
- Texts, videos and images should be preprocessed—for example, take characters like emojis or URLs out of text data, and resize videos to a consistent resolution.
Analysis: Once your data has been collected, cleaned and prepared, it’s time to feed it into your AI analysis tool of choice so it can detect patterns, correlations and anomalies you may not have caught otherwise.
Some common AI analysis techniques are:
- Descriptive analytics that summarize performance
- Predictive analytics that forecast future performance (more on that later!)
- Prescriptive analytics that recommend specific actions
- Natural Language Processing (NLP) which analyzes text content for different things like overall sentiment
- Computer vision which analyzes visual content
4. Visualization and interpretation
Many AI content analysis tools can present their findings in visual form upon completing their analysis. They can create graphs, charts and other visual aids that help us better identify any trends or patterns they picked up on in the data. You can also visualize the results yourself if needed.
Once you have your visualized analysis, you can also ask for recommendations for improving content performance. Or, you can take the insights and set your own actionable recommendations based on the findings.
Want to learn more about AI in the marketing industry? Check out our podcast episode where Naomi, Keegan Gates, and Shaheen Samavati discuss how to best integrate AI into the content creation process.
Predictive analytics: Planning future content with AI
Predictive analysis, as mentioned in the last section, is one of the most common types of content performance analysis. In a nutshell, predictive analysis uses a combination of provided data, statistical algorithms and machine-learning techniques to identify the probability of future outcomes.
Being able to leverage predictive analysis effectively can potentially be a game-changer. Using insights from historical data, AI can predict what kinds of topics or content will interest audiences in the near future. This way, you can be more proactive in your content creation, instead of just chasing after current trends. Plus, the more data you train your model with, the more effective it will be.
Predictive analytics has plenty of use cases in content marketing, as it can:
- Segment target audiences and anticipate the behavior of those segments.
- Optimize your resources by predicting the best times to run campaigns and choosing the most appropriate channels and audiences to target.
- Study the purchase history of consumers and help you create targeted campaigns based on preferences.
- Identify potential risks and issues before they escalate.
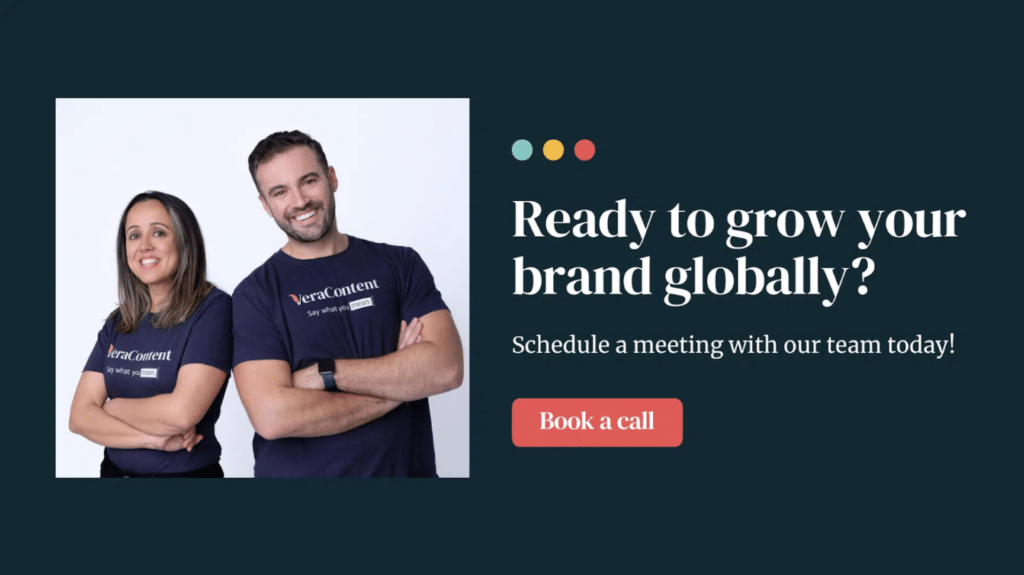
In addition to the AI content analysis steps described above, the most important steps you have to take when performing predictive analysis are defining the problem and choosing the predictive analysis technique you want to use. While you should approach all content analysis with a specific goal in mind, in predictive analysis it’s especially important, as this informs which technique is most effective.
Some of the most popular models used in prediction analysis:
Binary classification: This model assigns input data into two set categories. You could use this model to predict things like whether a recipient will open an email or not, whether something will go viral or not, or whether a customer will unfollow an Instagram account or not.
Multi-class classification: Similar to binary classification, this model assigns input data into more than two set categories. You could use it to predict which content format a user is more likely to engage with, sentiment trends, and which localized version of a piece of content will resonate best with different audiences.
Linear regression: One of the most commonly used predictive models, it can predict the relationship between independent variable(s) (actions) and dependent variable(s) (results). You could use it to predict how certain content types affect conversion rates, social posts’ performance, and the likelihood of a lead becoming a customer.
Logistic regression: This is a type of linear regression that’s most useful when you’re dealing with binary outcomes, like yes/no or success/failure, and want to predict the likelihood of that event occurring. You could use it to predict whether or not someone will open an email, like a post, or purchase a product after watching a webinar.
Decision trees: This is a very popular and powerful model that creates a “tree” of decisions and results based on past data, and then uses that tree to predict an unknown outcome. Typically, a tree has a “root” question that then splits off into smaller and smaller branches until finally reaching a result or leaf node.
For example, a decision tree predicting the success of a piece of content could start with the question of content type, then if the content is a blog, move on to asking the length, and then finally the time of publishing.
You could use decision trees to predict things like how well a piece of content will perform based on multiple variables, categorize leads based on their likelihood to convert to prioritize targeting the most promising prospects, and identify which content topics will best resonate with a certain audience given past preferences.
Random forest: Random forests use a collection of decision trees to predict specific outcomes. Each tree makes its own prediction, and the model averages out all of the trees’ predictions to give you a final result. This technique reduces the chance of noise sample data affecting the model and can handle more complex data patterns.
You could predict similar things with random forests that you can with decision trees, like predicting which products users are likely to be interested in, the effectiveness of digital ad campaigns, and which keywords and SEO techniques will help content rank higher.
Neural networks: Designed to work like a human brain does, neural networks can predict things in a more complex way than typical linear regression models. Instead of just inputs and outputs, they have a “hidden layer” that contains complex functions that create predictors. They’re especially useful for tasks that require deep learning, but they can be resource-intensive and demand large data sets.
Neural networks can create more accurate and granular content segments, identify emerging content trends, and predict what elements like color scheme or video length will drive more engagement or conversion.
Drawbacks of predictive analysis
Of course, there are some drawbacks to be aware of when using predictive analysis. You shouldn’t start letting it make most of your marketing strategy decisions for you. At the end of the day, you’ll still need humans to verify your results from these models and interpret it into actual content strategy.
Naomi Bleackley, AI Specialist at VeraContent, emphasizes the importance of using predictive analysis as a starting point, rather than a definitive solution. “It’s useful, but always take it with a grain of salt, not what you absolutely must do for your brand,” she suggests. Predictive analysis can spark ideas or provide a foundation, but Naomi stresses that it’s crucial to do your own research and not solely rely on AI-generated insights.
Some other common problems in predictive analysis are:
- Inaccurate predictions caused by inadequate or low-quality data.
- AI is trained on biased and flawed data, so in sensitive areas, you should keep in mind that AI by default has hidden biases.
- Many models are complex and don’t explain to you how they arrive at a certain conclusion
- Not all models may comply with AI regulations and ethical standards.
- There can be a skill gap in the market. There’s a huge demand right now for people who can interpret predictive analytics results.
AI tools you should be using to analyze your content
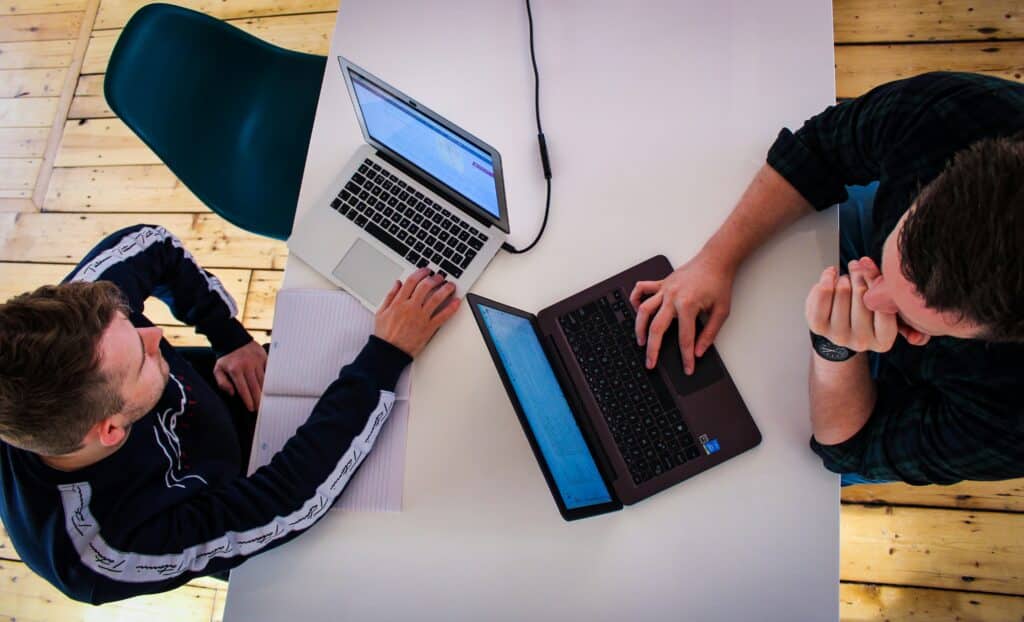
While you’re probably already using ChatGPT or another generative AI tool at work, there’s a world of AI tools out there, particularly when it comes to analyzing your content. While Chat GPT can work if you need it for content analysis, other tools may be more specialized for your goals.
Naomi explains that while ChatGPT is a very versatile and general-purpose tool—she says it’s the best tool to help with content creation—it may not be the best fit for a more specialized task like content analysis. “ChatGPT requires significant user input, custom prompts, and extensive testing to achieve specific goals,” she notes, adding that it doesn’t automatically sync with your data like many other dedicated tools.
Even if you implemented a custom GPT, Naomi explains, you’d have to either manually input a lot of data or set up something complex like API integration. In contrast, many specialized tools are already integrated within your social media platforms, CRMs, or other software, offering more seamless insight. “For tasks like this, using a purpose-built tool is often far more efficient and effective,” she says.
Best tools to help you with AI content analysis: Our top picks
Best for comprehensive analysis: Google Analytics
You probably already have a Google Analytics account, but did you know that it now has AI insights? Whether you’re tracking website performance or content engagement, Google Analytics 4 can help – and it’s probably already linked with your data.
Google Analytics leverages its AI capabilities in two different ways: predictive audiences and predictive metrics. Predictive audiences segment users based on their likelihood of doing a certain action, like making a purchase or subscribing. Predictive metrics can create segments based on predictive data, giving potential insight into how different segments can behave in the future.
To effectively use Google Analytics’s predictive metric tools, you have to meet certain criteria. The purchase event must be properly configured in your G4 property settings, so the AI can learn from user transactions. You’ll also need at least 1,000 samples of purchasers and 1,000 samples of non-purchasers or churned users, as the AI needs a substantial data set to learn from. If you meet these prerequisites, GA4 will calculate predictive metrics daily.
Best for content strategy: HubSpot
HubSpot has a whole suite of native tools to help boost your content. The HubSpot Content Hub can generate blog posts, social posts, and emails. It can also run A/B testing and analyze your content to help it align with audience interests and SEO best practices. Since many people already use HubSpot as a CRM, it can use your data to provide insights into your content’s performance and suggest topics your audience may be interested in.
HubSpot also has an AI “assistant” called Breeze that can help with content reporting. You can create even a single object report quickly using Breeze AI. When creating the report, you enter a phrase or question that summarizes what you want from the report, with object and time filters. Breeze sifts through all the data it has in the system and generates the relevant report.
Best for content optimization: MarketMuse
Market Muse is a powerful tool that uses AI to analyze your content quality, identify content gaps you (or your competitors) may have, and recommend improvements. It can even generate a content plan that pinpoints topic clusters that are shown to engage your target audience. It can look at already-published content to identify areas of improvement and increase your content score and ranking.
Best for market insights: GapScout
GapScout is a market research tool whose insights can be invaluable to your content marketing plan. Its AI can analyze reviews about your company and competitors, highlighting any gaps in the market. The information gained from this market research can give you ideas to refine ad copy and even identify new target segments, further optimizing your content strategy.
Success story: Using AI analysis to guide a global content strategy
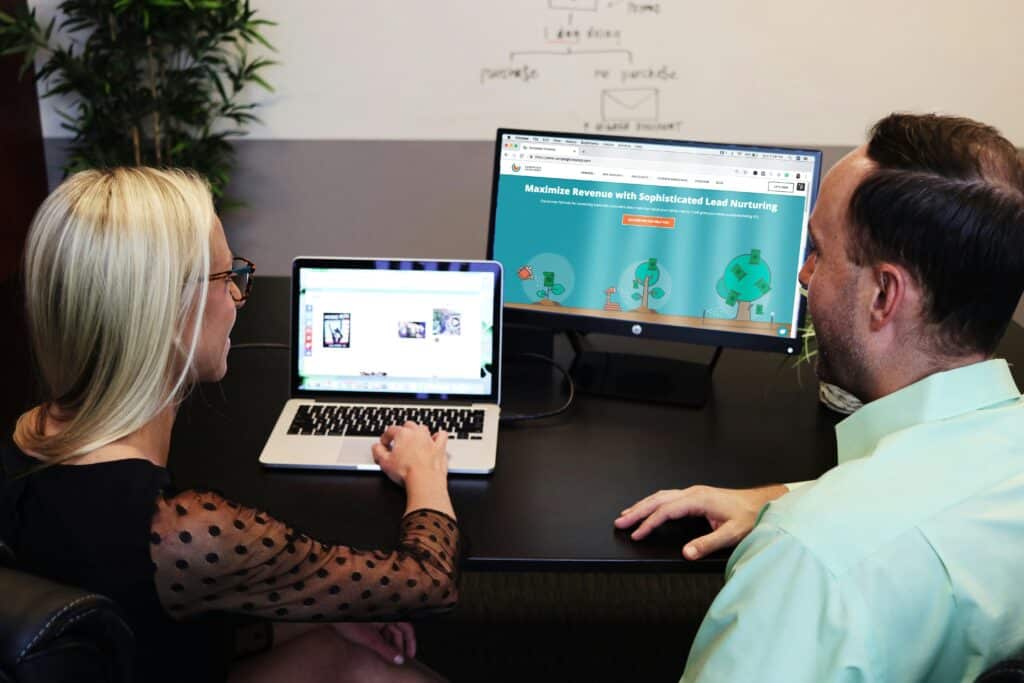
ClickUp, a project management software company, is relatively young, and in 2021 raised enough capital to expand its business to both the European and Asian Pacific markets. They quickly expanded their marketing team, who found themselves having to manage the performance of 500+ already existing articles and create new high-quality and SEO-optimized articles.
The team quickly turned to Surfer, an SEO content optimization platform that sports an AI-powered content intelligence feature. This tool does a lot – it can find topics most relevant to target audiences, develop content calendars, and analyze top SERPs to figure out what to target for favorable rankings.
In ClickUp’s case, content intelligence helped them identify high-value keywords, optimize content structure, and ensure articles met search intent. With the tool in place, ClickUp grew organic traffic by 85%, published over 150 blog posts, and completed 130 optimizations of already existing blog posts. One pillar article increased average rank from page 70 to the top of page two, and another post saw a traffic increase of 1334% after optimizations were implemented.
Our conclusion on using AI for multilingual and global content
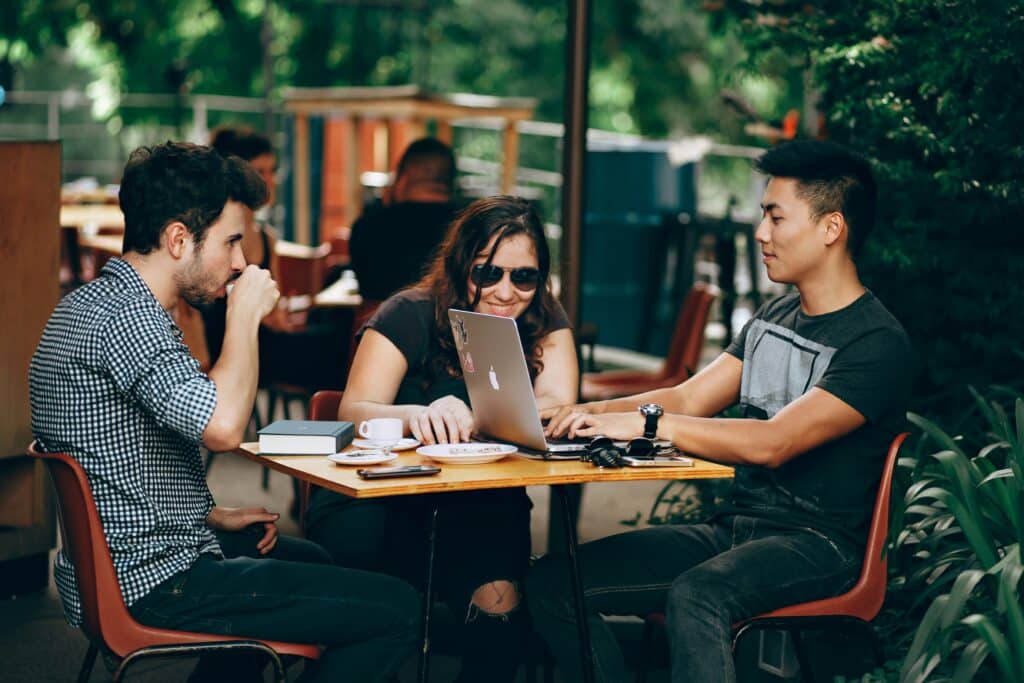
As we know, creating content in multiple languages is both time-consuming and expensive. While it’s the most accurate way to translate, for large volumes of content, human-led translation can take weeks or even months to complete. This is where AI can help.
One of the most used AI tools when creating multilingual content is machine translation. Most commonly used translation apps, like Google Translate, operate using machine translation. Thanks to advancements in deep learning and the increase in multilingual data available, these applications can produce more accurate and natural-sounding language, but they still make a lot of mistakes.
See also: Future of translation technology
NLP, or natural language processing, is the new powerhouse in AI-powered multilingual content. These models can help recognize patterns, syntax, context, and sentiment that can be missing from machine translation, making their translations more accurate and consistent across languages, instead of translating word-for-word.
Naomi, who also works as an English/Spanish translator, explained that she wrote a text full of complex slang and Gen-Z terms and tested it by running it through Google Translate, DeepL, and ChatGPT. She found that ChatGPT performed “the best by far, understanding the slang and picking up the nuances perfectly.”
See also: ChatGPT vs. machine translation tools
As a translator, Naomi prefers to work with AI over traditional machine translation, which she says often produces “gibberish” that makes no sense. While AI is still machine translation, it generates more natural-sounding text, even if it’s not completely accurate. “NLP translation still isn’t a perfect match for the original meaning, but at least it generates more fluent and coherent text,” she said.
NLP is a great tool to analyze multilingual content, particularly if no one on your team speaks a target language. It can analyze content in multiple languages to detect campaign sentiment in different regions, identify recurring topics or trends about your brand in UGC, and compare translations and localized content to make sure that translated content is still conveying the same core message.
It can also help identify relevant topics and keywords, analyze multilingual content for cultural sensitivity, adapt the content in real-time with dynamic translation and personalization, identify key entities like people, brands, and locations in text data, and compare campaign performance across regions to gauge content effectiveness and optimize resource allocation.
For example, a clothing company that operates in 15 languages discovers through sentiment analysis that its global slogan is seen as acceptable in North America and outdated in Europe. Using that information, the company creates a new slogan for the European market and increases customer engagement and conversion.
NLP has already been integrated into many online channels. Social media networks like Instagram use NLP to automatically translate UGC, like posts and comments, into a ton of local languages. Apps like Google Meet and Zoom now offer real-time captions and translation during video calls, so people can communicate with a much smaller language barrier. And Spotify is piloting a program that can translate podcasts into multiple languages using the podcaster’s voice.
Of course, there are still many challenges when using machine translation, even if the tools may be more sophisticated than before. Languages like English and Chinese have more content to train AI models on than other languages, which can bias AI towards these languages. Cultural biases present in all languages can also lead to incorrect translations and misunderstandings, and AI still needs help with nuances, idioms, and context-specific content.
Naomi emphasizes that “You should always work with a human translator in some capacity if your content is going to be published to represent your brand.” She says that for internal or personal documents, AI tools could be enough as that content isn’t public-facing. “But any content intended for a wider audience should always be reviewed by a human—even if it seems simple,” she said.
She points out that many AI tools, while sophisticated, can produce unexpected or inaccurate results called hallucinations. If no one on your team is a speaker of a target language, you may miss this completely and risk publishing content that can confuse or even offend your target market. “Human oversight is essential to ensure your final output is always reliable and polished,” she adds.
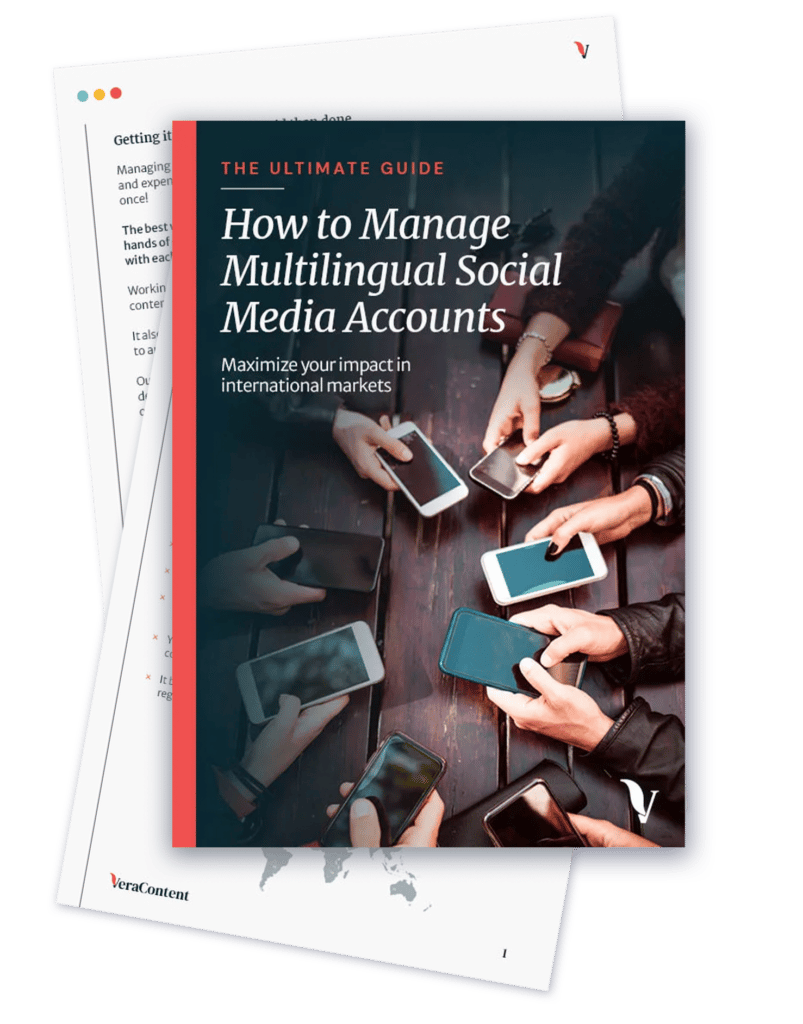